Requirements for AI-based Learning Experience Platforms (LXP)
1. Introduction
Learning Experience Platforms (LXP) constitute the backbone of modern corporate learning, particularly with their integration of artificial intelligence (AI). This article takes an in-depth look at the quality requirements of LXPs, focusing on ethical, legal, and moral aspects, as well as the control of training datasets.
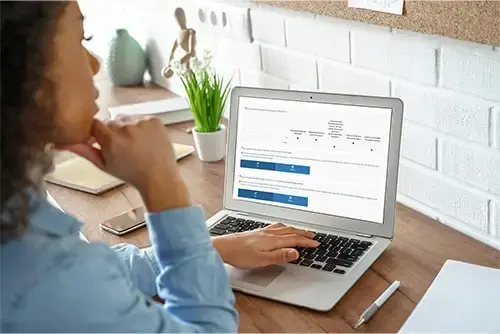
2. Objectives of Learning Experience Platforms (LXP)
The objective of LXPs extends beyond mere knowledge transfer, addressing the individual needs of both learners and organizations. For learners, this translates to a tailored learning experience that adapts to their specific skills, preferences, and learning goals. This is achieved through the application of AI algorithms generating personalized learning paths and responding to individual progress.
For organizations, especially for training administrators and professional development, LXPs offer new avenues for efficiency and optimization. The platforms enable precise analysis of learning behavior and provide insights into the effectiveness of training programs. Training administrators can thus respond to the needs of employees more effectively, and professional development benefits from improved talent management and the ability to strategically steer individual employee development.
3. How does a LXP work?
Operation of LXPs
Learning Experience Platforms (LXPs) leverage artificial intelligence (AI) to personalize learning experiences. Using algorithms, they analyze individual learning behaviors to create tailored learning paths and content. This allows for flexible adaptation to the needs and abilities of each learner. By incorporating Natural Language Processing (NLP), LXPs can even understand and respond to natural interactions, enhancing the overall learning experience.
Training Datasets
The effectiveness of LXPs relies on training datasets that serve as the foundation for AI algorithms. These datasets encompass a variety of information, including learning history, preferences, and learner feedback. It is crucial that these training datasets are diverse, representative, and free from systemic biases. Through careful selection and regular updates of this data, LXPs ensure precise customization to individual learning needs and avoid potential biases in recommended learning content.
The transparent handling of these training datasets is a fundamental aspect of ethical LXP development. Users should be informed about the use of AI to build trust while simultaneously protecting their privacy. The meticulous care and control of this data thus form the basis for high-quality and ethical LXP usage.
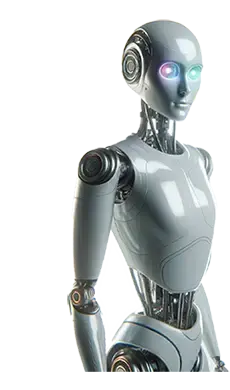
Example of a possible training dataset in the context of language learning
Case: "Learner A frequently engages with vocabulary related to technology and programming languages during evening study sessions."
Possible System Deductions:
-
Interest Identification:
- The system may deduce that Learner A has a keen interest in technology and programming languages based on their consistent engagement with related vocabulary.
- The system may deduce that Learner A has a keen interest in technology and programming languages based on their consistent engagement with related vocabulary.
-
Learning Preferences:
- The system could infer that Learner A prefers studying in the evening, indicating a potential peak learning time.
- The system could infer that Learner A prefers studying in the evening, indicating a potential peak learning time.
-
Adaptive Content Recommendation:
- With this information, the system might tailor future content recommendations to include more advanced or specialized topics in technology and programming languages for Learner A.
- With this information, the system might tailor future content recommendations to include more advanced or specialized topics in technology and programming languages for Learner A.
-
Study Session Optimization:
- The system could suggest optimizing study sessions by incorporating interactive coding exercises or quizzes related to the identified interests.
- The system could suggest optimizing study sessions by incorporating interactive coding exercises or quizzes related to the identified interests.
This example illustrates how a training dataset, comprising such sentences, helps the system understand individual learning patterns, preferences, and interests. The system can then use this information to provide a personalized and effective learning experience for the user.
4. The Evolution of LXP
4.1 Technological Advances and AI Integration
Rapid technological advances and seamless integration of AI have propelled LXP development. The application of Natural Language Processing (NLP) and machine learning allows for precise customization of learning content to individual needs.
4.2 Challenges in LXP Development
Despite the potential for innovation, developers face challenges such as handling large datasets, avoiding algorithmic biases, and ensuring data privacy.
It is important to note that the exact technological developments, ethical standards, and legal requirements may vary depending on the time and region. The description of the current situation is based on general principles and best practices at the time of writing this article.
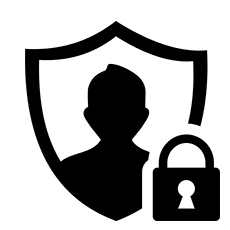
5. Quality Requirements for Training Datasets
5.1 Data Privacy in Training Datasets
Data privacy is paramount. This includes not only anonymizing personal data in training datasets but also implementing robust encryption technologies. An example of this is the pseudonymous storage of learning history data to protect the privacy of learners.
Additionally, clearly defining access rights is crucial. Learners should have the ability to individually adjust and control their privacy settings. Training portals can integrate this functionality.
5.2 Fairness and Avoidance of Systemic Biases
To ensure an inclusive learning environment, it is essential to scrutinize training datasets for systemic biases. A practical example is introducing diversity indicators when selecting training data to ensure algorithms do not unintentionally reproduce biases.
6. Ethics, Law, and Morality in LXP Development
6.1 Ethics as a Guiding Principle
The integration of ethical principles must be anchored as a guiding principle in LXP development. This could be achieved through the establishment of ethics committees regularly reviewing the impact of LXPs on learners and society as a whole.
6.2 Legal Aspects and Compliance
Compliance with legal provisions is an essential protective mechanism. A concrete example would be regular training of development teams on current data protection laws and regulations to ensure LXP systems are always in compliance.
6.3 Moral Principles in AI Development
Consideration of moral principles requires a proactive approach. A practical example could be creating guidelines for handling ethically sensitive learning content to ensure LXP platforms respect values and norms.
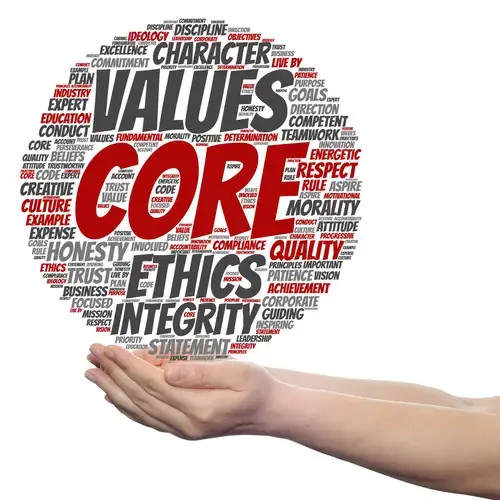
7. Ensuring Ethical LXP Development
7.1 Control and Documentation of Training Datasets
Detailed control and documentation of training datasets are crucial. This could involve implementing logging mechanisms that track the entire lifecycle of a training dataset, from data collection to application in algorithms.
7.2 Transparency as a Key Principle
Transparency plays a crucial role. An example could be the development of dashboards providing learners insight into the decision-making processes of algorithms to build trust and uncover potential biases.
7.3 Review and Evaluation of Models
Regular reviews and evaluations of AI models are necessary. An example would be the implementation of automated tests that not only check technical functionality but also assess ethical criteria and provide feedback for potential improvements.
8. Correction of Undesirable Results
8.1 Identification of Errors and Biases
Identifying undesirable results requires systematic review. An example would be conducting regular audits where specialized teams identify and document potential errors and biases in the algorithms.
8.2 Adaptive Adjustments and Continuous Improvement
The ability to respond to undesirable results requires adaptive adjustments. An example could be the integration of feedback loops used by learners and trainers to report shortcomings, enabling continuous improvements.
9. Conclusion
Striking the optimal balance between technological advancement and ethical responsibility is crucial for creating high-quality and fair learning experiences. It is important to note that the exact technological developments, ethical standards, and legal requirements may vary depending on the time and region. The description of the current situation is based on general principles and best practices at the time of writing this article.
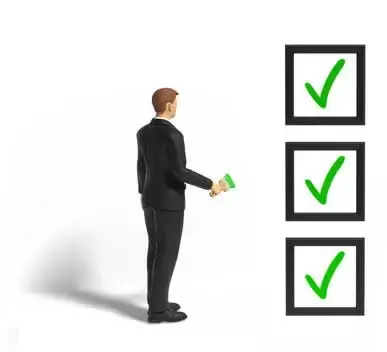
Checklist for Training Administrators and Professional Development
Verify Data Privacy:
- Protect sensitive data in training datasets, e.g., through anonymization and encryption.
- Provide control and settings for access to personal data.
Ensure Fairness:
- Regularly check for systemic biases through targeted analyses.
- Make adjustments to ensure fairness in LXP algorithms.
Expected Benefits for Learners:
- Personalized learning experience for individual needs and learning goals.
- Flexibility in learning paths and pace.
Expected Benefits for Organization:
- Efficient resource utilization through targeted assignment of qualification paths.
- Precise analysis of learning behavior for strategic talent management.
Consider Ethics and Law:
- Establish clear guidelines for ethical behavior and regularly review compliance.
- Implement emergency mechanisms to address unforeseen ethical dilemmas.
Establish Transparent Processes:
- Clearly log training datasets for transparency and traceability.
- Provide notifications to learners about the use of AI algorithms.
Continuous Evaluation:
- Regularly review and evaluate AI models.
- Implement mechanisms for automated model monitoring.
Prompt Intervention for Undesirable Results:
- Conduct systematic audits to identify errors and biases.
- Implement adaptive adjustments and ensure continuous training of models.
The principles and recommendations described offer a comprehensive perspective on the quality requirements of LXPs. However, it is strongly recommended to consider current developments, specific legal requirements, and industry-specific recommendations. It is advisable to conduct further research to ensure the information is up-to-date.
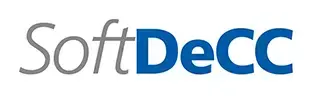
About us
Since 1998 SoftDeCC is working closely with major training centers and academies. This results in a unique experience with training requirements.
Our Learning Management System is designed to adjust to individual corporate learning processes and address evolving challenges. More...
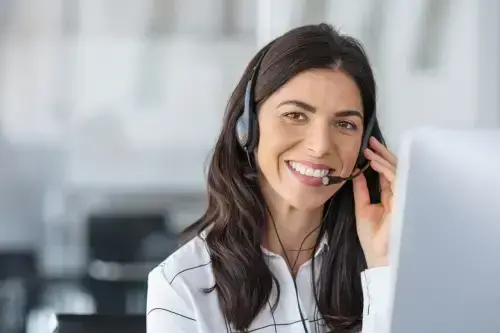
Speak to us
We are looking forward to discussing your learning challenge with you and give you a free and personal guided tour of our management software.
Call +49 (0)89 / 3090 839 30.
Also interesting for you:
Learn more about our LXP-offer